일 | 월 | 화 | 수 | 목 | 금 | 토 |
---|---|---|---|---|---|---|
1 | ||||||
2 | 3 | 4 | 5 | 6 | 7 | 8 |
9 | 10 | 11 | 12 | 13 | 14 | 15 |
16 | 17 | 18 | 19 | 20 | 21 | 22 |
23 | 24 | 25 | 26 | 27 | 28 |
- pycharm
- 결합전문기관
- CES 2O21 참가
- bccard
- 머신러닝
- C언어
- postorder
- paragraph
- html
- inorder
- web 사진
- tensorflow
- java역사
- 자료구조
- vscode
- 데이터전문기관
- mglearn
- broscoding
- 웹 용어
- classification
- Keras
- 재귀함수
- 대이터
- CES 2O21 참여
- discrete_scatter
- cudnn
- web
- web 용어
- web 개발
- KNeighborsClassifier
- Today
- Total
목록머신러닝 (3)
bro's coding
import numpy as np import pandas as pd import matplotlib.pyplot as plt from sklearn.datasets import load_breast_cancer cancer =load_breast_cancer() X=cancer.data y=cancer.target from sklearn.model_selection import train_test_split X_train,X_test,y_train,y_test=train_test_split(X,y) from sklearn.svm import SVC model=SVC() model.fit(X_train,y_train) model.score(X_test,y_test) # 0.6083916083916084 ..
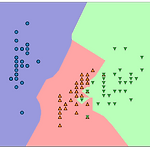
import mglearn model=KNeighborsClassifier(n_neighbors=1) # n_neighbors=1 model.fit(X_train,y_train) plt.figure(figsize=[10,8]) # 그래프 사이즈 mglearn.plots.plot_2d_classification(model,X_train,fill=True,eps=0.5,alpha=0.4) # 바탕색과 관련 mglearn.discrete_scatter(X_train[:,0],X_train[:,1],y_train) # train data에 대한 산점도 #mglearn.discrete_scatter(X_test[:,0],X_test[:,1],y_test) # test data에 대한 산점도 plt.figure(f..
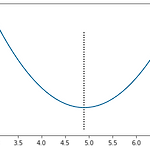
stochastic gradient descent rng=np.arange(mm-2,mm+2,0.1) plt.plot(rng,((data-rng.reshape(-1,1))**2).mean(axis=1)) plt.vlines([mm],11,15,linestyles=':') plt.ylabel=('MSE') plt.xlabel=('prediction') # 평균일 때, 오차가 가장 작고 # 평균과 멀어지면 오차는 증가한다.