일 | 월 | 화 | 수 | 목 | 금 | 토 |
---|---|---|---|---|---|---|
1 | ||||||
2 | 3 | 4 | 5 | 6 | 7 | 8 |
9 | 10 | 11 | 12 | 13 | 14 | 15 |
16 | 17 | 18 | 19 | 20 | 21 | 22 |
23 | 24 | 25 | 26 | 27 | 28 |
- CES 2O21 참가
- paragraph
- web
- 결합전문기관
- vscode
- discrete_scatter
- Keras
- 웹 용어
- C언어
- cudnn
- classification
- postorder
- inorder
- KNeighborsClassifier
- tensorflow
- bccard
- web 개발
- 머신러닝
- web 용어
- mglearn
- 자료구조
- html
- CES 2O21 참여
- java역사
- broscoding
- 대이터
- pycharm
- 데이터전문기관
- web 사진
- 재귀함수
- Today
- Total
목록[CERTIFICATION] (21)
bro's coding
https://jsw6701.github.io/ai/1 [AI] AICE Associate 시험 내용 및 데이터 전처리, 머신러닝, 딥러닝 jsw6701.github.io
딥러닝Permalink 라이브러리 importPermalink import tensorflow as tf from tensorflow.keras.models import Sequential from tensorflow.keras.layers import Dense, Dropout tf.random.set_seed(100) Sequential 모델 생성Permalink # Sequential() 모델 정의 하고 model로 저장 # input layer는 input_shape=() 옵션을 사용한다. # 39개 input layer # unit 4개 hidden layer # unit 3개 hidden layer # 1개 output layser : 이진분류 model = Sequential() model...
데이터 정규화/스케일링(Normalizing/Scaling)Permalink from sklearn.preprocessing import MinMaxScaler scaler = MinMaxScaler() X_train = scaler.fit_transform(X_train) X_test = scaler.transform(X_test)
Tranin, Test 데이터셋 분할Permalink 입력(X)과 레이블 (y) 나누기 = df1.drop('Churn', axis=1).values y = df1['Churn'].values from sklearn.model_selection import train_test_split X_train, X_test, y_train, y_test = train_test_split(X, y, test_size=0.3, stratify=y, random_state=42) 모델 개발Permalink 모델별 바차트 그려주고 성능 확인을 위한 함수 # 모델별로 Recall 점수 저장 # 모델 Recall 점수 순서대로 바차트를 그려 모델별로 성능 확인 가능 from sklearn.metrics import accu..
오브젝트 타입 골라서 → 전부 숫자형Permalink LabelEncoder 활용 : Pandas get_dummies 함수 이용하여 One-Hot-Encoding cal_cols = df.select_dtypes('object').columns.values df1 = pd.get_dummies(data=df, columns=cal_cols)
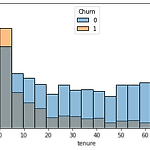
시각화Permalink 라이브러리 import import matplotlib.pyplot as plt %matplotlib inline Bar Chart df[’gender’].value_counts().plot(kind=’bar’) Object 컬럼 하나씩 가져와서 Bar 차트 그리기 object_list = df.select_dtypes('object').columns.values for col in object_list: df[col].value_counts().plot(kind='bar') plt.title(col) plt.show() Histgram import seaborn as sns sns.histplot(data=df, x=’tenure’, hue=’Churn’) 곡선 히스토그램 : s..
필요 라이브러리 임포트 import numpy as np import pandas as pd #파일 읽어오기 df = pd.read_csv(’data_v1.csv’) #탐색적 데이터 분석 #상위 디폴트 5개 : df.head() #하위 디폴트 5개 : df.tail() #자료구조 파악 : df.info() #데이터 인덱스 : df.index #데이터 컬럼명 : df.columns #데이터 Values : df.values #Null 데이터 확인 : df.isnull().sum() #통계 정보 : df.describe() #데이터 전처리 #자료 구조 파악 info 사용(Row, column, Not-null, type) #customerID는 필요 없으니 삭제 df.drop(’customerID’, axi..
setup( 아이템 기획, 팀빌딩) - 요구분석 - 설계 - 구현 - 테스트 -이행 -운영(유지보수) > 마일스톤 >폭포수 >애자일 : 반복 점증
요구사항 분석 > 설계 > 구현 > 테스트 > 운영
요구사항분석 > 설계 > 구현 > 테스트 > 이행 > 유지보수