반응형
Notice
Recent Posts
Recent Comments
Link
일 | 월 | 화 | 수 | 목 | 금 | 토 |
---|---|---|---|---|---|---|
1 | 2 | 3 | 4 | 5 | ||
6 | 7 | 8 | 9 | 10 | 11 | 12 |
13 | 14 | 15 | 16 | 17 | 18 | 19 |
20 | 21 | 22 | 23 | 24 | 25 | 26 |
27 | 28 | 29 | 30 | 31 |
Tags
- web 사진
- web 개발
- broscoding
- vscode
- 자료구조
- 대이터
- 웹 용어
- 재귀함수
- cudnn
- tensorflow
- inorder
- web 용어
- KNeighborsClassifier
- discrete_scatter
- postorder
- classification
- mglearn
- 데이터전문기관
- pycharm
- paragraph
- C언어
- 결합전문기관
- 머신러닝
- java역사
- Keras
- web
- CES 2O21 참여
- CES 2O21 참가
- bccard
- html
Archives
- Today
- Total
bro's coding
keras.basic(예측) 본문
반응형
import numpy as np
import keras
from keras.models import Sequential
from keras.layers import Dense
x=np.array([1,2,3])
y=np.array([3,5,9])
model =Sequential()
model.add(Dense(1,input_shape=(1,),activation='linear'))
# 1 : 중간층 없음
# input_shape=(1,) : 속성 갯수
# activation='linear') : 활성 함수
#학습률 지정안함
#가중치 초기화 안함
model.compile(loss='mse',optimizer='sgd')
# loss='mse' : 비용함수
# optimizer='sgd' : 옵티마이져
model.fit(x.reshape(-1,1),y,epochs=1000)
# x.reshape(-1,1),y : 훈련 data
# epochs=1000 : 반복 횟수(에포크)
# 예측
model.predict(x.reshape(-1,1))
array([[2.7419074],
[5.682813 ],
[8.623719 ]], dtype=float32)
import matplotlib.pyplot as plt
plt.scatter(x,y)
plt.plot(x,model.predict(x.reshape(-1,1)).ravel(),'r:')
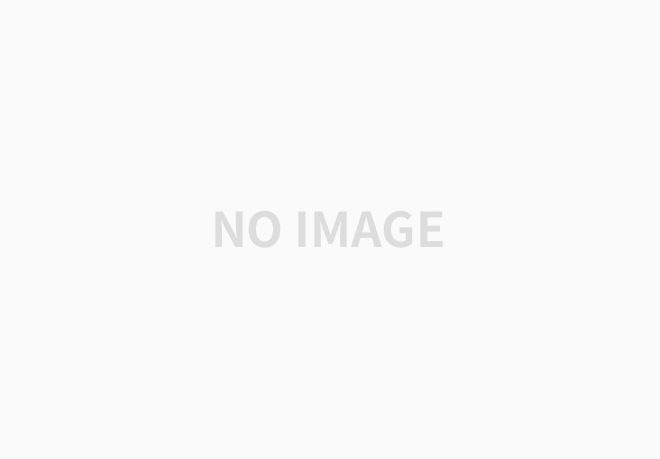
ws = model.get_weights() # 계산 된 가중치 값을 가져온다.(w,b)
ws
[array([[2.9409058]], dtype=float32), array([-0.19899847], dtype=float32)]
w=ws[0][0,0]
b=ws[1][0]
w,b
(2.9409058, -0.19899847)
h=model.history.history
plt.plot(h['loss'])
plt.ylim([0,1])
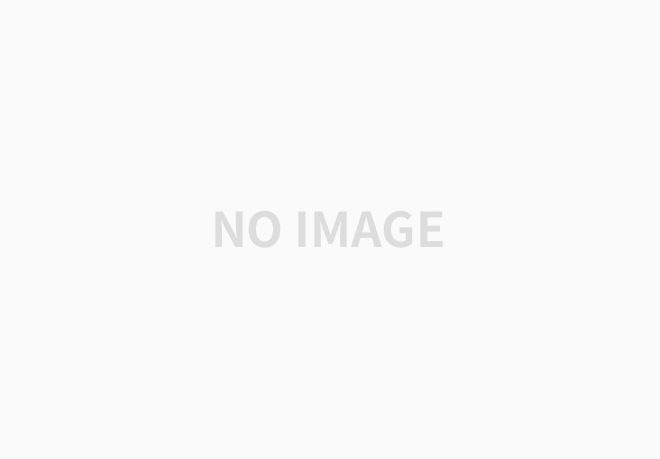
반응형
'[AI] > python.keras' 카테고리의 다른 글
keras.score (0) | 2020.05.13 |
---|---|
keras.mnist(중간층) (0) | 2020.05.13 |
keras.mnist(중간층X) (0) | 2020.05.13 |
keras.mnist (0) | 2020.05.13 |
keras.iris(분류) (0) | 2020.05.13 |
keras.basic(분류) (0) | 2020.05.13 |
keras.iris(예측 ) (0) | 2020.05.13 |
keras.install (0) | 2020.05.13 |